Someone mentioned cargo-bloat the other day and it reminded me that I have been wanting to measure the code size for generic functions in librsvg, and see if there are improvements to be made.
Performance
Optimisation, benchmarks, etc. Posts 
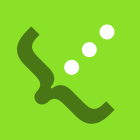
accessibilityIn our recent status update, we called out the use of a single large data structure for all accessible UI elements as a known potential weakness in the design. After that post, feedback from potential users of AccessKit made it clear that this design flaw was a pressing problem that blocked them from using AccessKit. One of these discussions also led us to a particularly attractive technique for solving the problem. So we decided to go ahead and do this optimization work, to unblock further adoption of AccessKit and get the inevitable incompatible API changes out of the way sooner rather than later. This post summarizes the results of this optimization, explains how we did it, and looks ahead to further potential optimizations.
This post describes work in progress: how cargo-semver-checks will benefit from the upcoming query optimization API in the Trustfall query engine. Read on to learn how a modern linter works under the hood, and how ideas from the world of databases can improve its performance.
I have recently discovered that Rust Futures, or rather, async fn calls can lead to surprising performance problems if they are nested too deeply.
Apart from highlighting the source of the problem in great depth, I also want to propose some workarounds for this specific issue.
android simd armThis blog post is the last one of a series exploring SIMD support with Rust on Android. In the previous two posts, I introduced how to compile Rust libraries for Android and detect SIMD instructions supported by the CPU at runtime.
Today, we’ll see how to effectively use the SIMD instructions themselves, and get the most performance out of them. After an introduction on running Rust benchmarks (and unit tests) on Android devices, we’ll measure the performance in various scenarios offered by Rust, and see that the overhead of CPU feature detection can be non-trivial. I’ll then describe various ways to reduce this overhead.
Lastly, I’ll present updated benchmarks on ARM of Horcrux, my Rust implementation of Shamir’s Secret Sharing, and see how they compare to Intel.
C++ is notorious for its slow build times. “My code's compiling” is a meme in the programming world, and C++ keeps this joke alive.
I've heard the same thing about Rust: build times are a huge problem. But is it really a problem in Rust, or is this anti-Rust propaganda? How does it compare to C++'s build time problem?
Rust prevents out-of-bounds memory accesses and buffer overruns via runtime bounds checks - what’s the cost of those bounds checks for a real-world, production application?
One of the nice things about the Rust programming language is that it makes it easier to write correct concurrent (e.g. threaded) programs – to the degree that Rust’s slogan has been, at times, “fearless concurrency.”
But I’d like to tell you about the other side of Rust, which I think is under-appreciated. Rust enables you to write programs that are not concurrent. This feature is missing from most other languages, and is a source of much complexity and bugs.
Hi everyone! @GuillaumeGomez recently tweeted about the rustdoc performance improvements and suggested that we write a blog post about it:
> The performance comparison between @rustlang rustdoc now and rustdoc 4 months ago are crazy! The rustdoc cleanup going on (we're still far from done!) is having a huge positive impact! Can't wait to see how much better the results will be. Maybe I should write a blog post? pic.twitter.com/XapdmdZ1IZ — Guillaume Gomez (@imperioworld_) January 13, 2021
The tweet received a lot of comments approving the blog post idea so here we go!
For the last few months Mozilla has been using Profile-Guided Optimization (PGO) to build their own optimized version of Clang, leading to an up to 9% reduction of Firefox compile times on their build infrastructure. Would the same be possible for the Rust compiler, that is, could we apply profile-guided optimization to rustc itself in order to make it faster? This post explores exactly this question, detailing first the steps needed for generating a PGOed versions of rustc (in two flavors), and then taking a look at the resulting performance implications. But before that let's have a little reminder what PGO even is and how it works in general.
This article will explore the thread-per-core model with its advantages and challenges, and introduce Scipio (you can also find it on crates.io), our solution to this problem. Scipio allows Rust developers to write thread-per-core applications in an easy and manageable way.
Back in spring 2020 at GoOut, we were looking to replace our Spring-Tomcat duo by a more lightweight framework to power our future Kotlin microservices. We did some detailed (at times philosophical) theoretical comparisons that I much enjoyed, but these cannot substitute a hands-on experience. We decided to implement proof-of-concept microservices using the most viable frameworks, stressing them in a benchmark along the way. While Kotlin was the main language, I saw this as an opportunity to have some fun at home and test (my proficiency with) Rust, which is touted for being fast. I ended up stress-testing Kotlin’s Http4k, Ktor, and Rust’s Actix Web, read on to see how they fared.
In the previous post, I showed how processing file data in parallel can either boost or hurt performance depending on the workload and device capabilities. Therefore, in complex programs that mix tasks of different types using different physical resources, e.g. CPU, storage (e.g. HDD/SSD) or network I/O, a need may arise to configure parallelism levels differently for each task type. This is typically solved by scheduling tasks of different types on dedicated thread pools. In this post I’m showing how to implement a solution in Rust with Rayon.
Not all profiling experiences are alike. Some are filled with friction around the tooling. Others are around doubt about whether or not intermediate layers are inflating or shifting numbers in unfair ways. Perhaps you work in a security or data-compliance critical environment and all you want is numbers on what is running in production without having to breach agreements by downloading live data to your work environment. Benchmarks are fantastic for tracking numbers of common or pathological cases over time, but they may still be unrealistic in comparison to the undiscovered cases in prod. When I find that I can't easily wedge in a profile, I get a bit sad and then turn to crude solutions.
I've had the pleasure to work with very experienced firmware developers; the kind of people who know the size of their red zones and routinely transform coffee into linker scripts and pointer dereferences. In other words, the Mels and Zeus Hammers of the world.
When it comes to the tools of our trade, many of them are curious and experimental. Some of them—very much myself included—explore far enough to leave pragmatism behind and veer into idealism, stubbornly forcing beautiful round pegs into industrial square holes. Hey, maybe they're square for a reason, but it doesn't hurt to try.
The majority of them aren't like that. Your average battle-tested firmware developer has accrued a healthy distrust of the abstract, probably born of watching shiny platonic constructs crash and burn with painfully real and concrete error traces. It is sobering, having to chase a hardfault on a tiny MCU across enough vtables and templated code to make Herb Sutter puke angle brackets. No wonder modern approaches are met with some resistance unless the LOADs and the STOREs are in clear view.
I felt this way too when someone suggested to me, back in 2014, that an up-and-coming language called Rust showed promise in the embedded field. Surely not, I thought, too high level. Even though I had been playing with it already, my profoundly ingrained bit-twiddling instincts told me not to trust a language that supported functional programming, or one that dared to have an opinion on how I managed my memory. Bah! That's how you get philosophers to run out of forks, and your forks to turn into SIGSEGVs.
I was wrong.
This might be a bit of an unpopular opinion, so the clickbait title should be appropriate!
I've been learning and using Rust for nearly four and a half years, since version 1.6. That's a good amount of time to become familiar with some of the troubles of the language, the tooling, and the ecosystem. But this is a slightly different story, this is a dissent to a common criticism that the compiler is slow. In this discussion, I will argue that these claims are misleading at best.
At Bearer, we are a polyglot engineering team. Both in spoken languages and programming languages. Our stack is made up of services written in Node.js, Ruby, Elixir, and a handful of others in addition to all the languages our agent library supports. Like most teams, we balance using the right tool for the job with using the right tool for the time. Recently, we reached a limitation in one of our services that led us to transition that service from Node.js to Rust. This post goes into some of the details that caused the need to change languages, as well as some of the decisions we made along the way.
This guide covers timeless ideas that are helpful to keep in mind while working with systems where performance matters. Many of these ideas are fairly “durable” and will apply regardless of what hardware, programming language, operating system, or decade you are working in.
Stay tuned for follow-up articles that will drill into hardware and more Rust-specific techniques.
A compelling feature of Rust and C++ is "zero-cost abstractions". You can write "high level" code, e.g. using iterators, that compiles down to the same machine code as the low-level code that you'd write by hand. You can add layers of abstraction, e.g. wrapping a primitive value in a struct and providing a specialized API for it, without adding run-time overhead. However, "zero-cost" only applies if you enable an adequate set of compiler optimizations. Unfortunately, enabling those optimizations slows down compilation and, with current compilers, trashes a lot of debug information, making it very difficult to debug with those binaries. Since the Rust standard library (and increasingly the C++ standard library) makes heavy use of "zero-cost" abstractions, using non-optimized builds for the sake of better debugging and build times creates binaries that are many times slower than release builds, often untenably slow. So the question is: how can we get fast builds and quality debuginfo while keeping zero-cost abstractions?
Most Rust programmers have heard of Rayon, a crate that makes it almost magically easy to introduce parallelism to a program. In this article we’ll examine how to apply Rayon to basic stream processing.
I last wrote in April about my work on speeding up the Rust compiler. Time for another update.
Weekly performance triageFirst up is a process change: I have started doing weekly performance triage. Each Tuesday I have been looking at the performance results of all the PRs merged in the past week. For each PR that has regressed or improved performance by a non-negligible amount, I add a comment to the PR with a link to the measurements. I also gather these results into a weekly report, which is mentioned in This Week in Rust, and also looked at in the weekly compiler team meeting.
The goal of this is to ensure that regressions are caught quickly and appropriate action is taken, and to raise awareness of performance issues in general.
pythonRust can be used to speed up Python code, but how fast can it be? This article highlights the comparison between the performance of: Rust, PyO3, and Python3, and an easy way to setup PyO3.
Data-oriented design is an approach to optimising programs by carefully considering the memory layout of data structures, and their implications for auto-vectorisation and use of the CPU cache. I highly recommend watching Mike Acton’s “Data-Oriented Design and C++” talk if you haven’t seen it already.
In this post we will cover 4 cases, using criterion for benchmarking.
As part of an Undergraduate Research Assistant Scheme in my first year of university I was tasked with parallelising a piece of shallow water simulation software written in FORTRAN by Dr David Dritschel of the Vortex Dynamics Research Group, under supervision of Dr. Alexander Konovalov, at the University of St Andrews. There were secondary goals such as improving the testing infrastructure, setting up CI/CD, estimating progress and allowing the computation to be paused and resumed.
Forewarning: I have essentially zero domain knowledge in this project (and fluid dynamics simulation isn’t exactly the kind of topic you can catch up to research level on over a weekend) so I approached this project from a purely software engineering perspective. As for my Rust experience, I’ve been using it for personal projects since ~2016 and I worked as a Rust software engineer at a startup in Berlin for a year after leaving high school.
asyncThe async/await keywords in modern Rust make building high-throughput daemons pretty straightforward, but as I learned that doesn’t necessarily mean “easy.” Last month on the Scribd tech blog wrote about a daemon named hotdog which we deployed into production: Ingesting production logs with Rust. In this post, I would like to write about some of the technical challenges I encountered getting the performance tuned for this async-std based Rust application.
audioI ported a C library to rust last week, and it went pretty smoothly. The library in question is RNNoise, a library for removing noise from audio. It works well, it runs fast, and best of all it has no knobs that you need to tune. There’s even a rust
binding.
If you had to parse a microsecond-resolution epoch timestamp as quickly as possible, how would you do it? We’ll take a look at using compiler intrinsics to do it in log(n) time.
simdIn the previous article on auto-vectorization we looked at the different SIMD instruction set families on X86-64. We saw how he target-feature compiler flag and #[target_feature()] attribute gave us more control over the instructions used in the generated assembly.
There is a related compiler flag target-cpu we didn’t touch on, so it’s worth taking a look at how it affects the generated code.
simdSince the last post about SIMD library plans, I’ve been experimenting. Needless to say, it turned out a bit different than originally planned, but I’ve something I’d like to share. Maybe it’ll be useful for someone or maybe it’ll at least spark some more progress in the area.
If you don’t care about the chatter and just want to use it, it’s called slipstream and is available on crates.io. It’s an early release and will need some more work, but it can be experimented with (it has documentation and probably won’t eat any kittens when used). If you want to help out, scroll down to see what needs help (or decide on your own what part needs improving 😇).
I dread running cargo build --release.
It's not that I dread build failures or compiler warnings - I dread the wait.
My current Rust project takes about a minute to build on my laptop. It's not long enough to do something meaningful, like read HackerNews, but is long enough to make it feel agonizingly boring. If I don't have the money for a faster laptop, maybe I could build a really fast Rust development server on AWS?
When it comes to runtime performance, Rust is one of the fastest guns in the west. It is on par with the likes of C and C++ and sometimes even surpasses them. Compile times, however? That's a different story.
This is a handy pattern to use for efficiently escaping text, and it's also a good demonstration of Rust's Cow 🐄 type.
asmWhat do some Rust features compile to? I have been starting to have a look at Rust lately, mostly because WASM is growing on me and Rust has the best tool in class for it, or so I am told. I am eager to find out by myself. Rust comes with several new idioms and structures in the language I am not used to, and being a performance enthusiast, I always get interested in what such constructs translate to.
simdIn the previous article on auto-vectorization we treated instructions as either SIMD (Single Instruction Multiple Data) or non-SIMD. We also assumed that SIMD meant four values at a time.
That was true for way we wrote and compiled our code in that article, but we're going to expand beyond that. There is a progression of SIMD instruction families, with new releases of CPU's from Intel and AMD supporting new instructions we can use to increase the performance of our code.
If our goal is to get the best performance we need to take advantage of all the possible SIMD instructions on our hardware.
In this article we're going to:
* Look at the compiler output when targeting the different SIMD instruction set families.
* Benchmark the different instruction sets.
* Look at how we can structure our Rust code to support compiling for multiple instruction sets and then selecting at runtime the one to use.
simdRecently on a project I wrote some audio processing code in Rust. In the past I've used C++ to write audio processing code for situations where performance was critical. I wanted to take that C++ optimisation experience and see what is possible using Rust.
We're going to take one small piece of audio processing code and take a look at how we can optimize it in Rust. Along the way we're going to learn about optimisation using Single Instruction Multiple Data CPU instructions, how to quickly check the assembler output of the compiler, and simple changes we can make to our Rust code to produce faster programs.
When working on Rust applications or CLIs that need to show something to the end user as fast as possible I often find that a significant chunk of the time is usually spent not in doing any computations, but in dropping large data structures at the end of the function.
A common complaint heard about Rust is the size of the binary it produces. They are various reasons explaining why Rust binaries are generally bigger that ones produced with lower level languages such as C. The main one is Cargo, Rust's package manager and building tool, producing static binaries by default. While larger binaries are generally not much of an issue for desktop or server applications, it may become more of a problem on embedded systems where storage and/or memory may be very limited.
GStreamer is used extensively at Collabora to help our clients to build embedded multimedia solutions. With Rust gaining traction among the GStreamer community as an alternative to C to write GStreamer applications and plugins, we began wondering if the size of such Rust plugins would be a problem for embedded systems, and what could be done to reduce sizes as much as possible.
Inspired by this Tiny Rocket analysis and the Minimizing Rust Binary Size repository, here are the different strategies we tried to reduce the size of a minimal Rust GStreamer plugin.
I last wrote in December 2019 about my work on speeding up the Rust compiler. Time for another update.
I recently undertook a project to improve the stack fixing tools used for Firefox. This has resulted in some large performance wins (e.g. 10x-100x) and a significant improvement in code quality. The story involves Rust, Python, executable and debug info formats, Taskcluster, and many unexpected complications.
Fast iteration times are something that many game developers consider to be of utmost importance. Keeping build times short is a major component of quick iteration for a programmer. Aside from the actual time spent compiling, any time you have to wait long enough that you start to lose focus on the activity you are working on, or you start to get distracted or lose track of what you were doing which costs you more time.
Thus one of my goals when writing glam was to ensure it was fast to compile. Rust compile times are known to be a bit slow compared to many other languages, and I didn’t want to pour fuel on to that particular fire.
As part of writing glam I also wrote mathbench so I could compare performance with similar libraries. I also always wanted to include build time comparisons as part of mathbench and I’ve finally got around to doing that with a new tool called buildbench.
In this post I'd like to introduce the next major change that will be released in nalgebra at the end of this month (March 2020). This change is about adding the support for SIMD AoSoA to nalgebra. I'll explain what I mean by SIMD AoSoA (Array-of-Structures-of-Arrays with explicit SIMD) and how it relates to SoA (Structure-of-Arrays) and AoS (Array-of-Structures). To give you an idea, SIMD AoSoA is actually what the recent ultraviolet crate has been using to achieve its amazing performances.
Let's continue with the enormous SVG from the last time, a map extracted from OpenStreetMap. According to Massif, peak memory consumption for that file occurs at the following point during the execution of rsvg-convert.
wasmIn my last post I mentioned that pa’i was faster than Olin’s cwa binary written in go without giving any benchmarks. I’ve been working on new ways to gather and visualize these benchmarks, and here they are.
Benchmarking WebAssembly implementations is slightly hard. A lot of existing benchmark tools simply do not run in WebAssembly as is, not to mention inside the Olin ABI. However, I have created a few tasks that I feel represent common tasks that pa’i (and later wasmcloud).
To collect profiling statistics for Rust programs like TiKV, we developed pprof-rs, which samples, analyzes, and visualizes performance data in one step. We integrated pprof-rs in TiKV's status_server, which outputs monitoring information.
Because pprof-rs uses the same data format as the Go tool pprof, we can use pprof, to visualize TiKV's profiling data. This makes it easier for developers and online users to find TiKV's performance bottlenecks.
We got a bug with a gigantic SVG of a map extracted from OpenStreetMap, and it has about 600,000 elements. Most of them are <path>, that is, specifications for Bézier paths.
To continue with last time's topic, let's see how to make librsvg's DOM nodes smaller in memory. Since that time, there have been some changes to the code; that is why in this post some of the type names are different from last time's.
In this post we are going to explore how to try and create an embedded version of the Mender-Rust binary which we created in the project from the previous post in this series. The original goal is to get it below 1 MB, but this sounds a bit ambitious to me. Let us see how we fare in the world of binary minimization.
A few years back, I read a quite unique blog about C++. It was actually one of the first times I was exposed to the concept of technical blogs. And with it the whole concept of learning software development outside the education system (e.g., the good old courses, exercises and sitting in a class being taught by a teacher). It is, to the date, one of my favorite blog posts and a cornerstone in my desire to write my own blog.
The blog was written by Herb Sutter, more than 20 years ago, and you can find it here. Before discussing the blog and why it is still relevant, more than 20 years later, we need some background. It describes an optimization known as Copy-On-Write, abbreviated as COW. I’m going to cover this optimization both in Rust and C++.
Librsvg's memory consumption has not been a problem so far for GNOME's use cases, which is basically rendering icons. But for SVG files with thousands of elements, it could do a lot better.
So you’re writing Rust but it’s not fast enough? Even though you’re using cargo build --release? Here’s some small things you can do to increase the runtime speed of a Rust project – practically without changing any code!
Please remember that the following suggestions do not replace actual profiling and optimizations! I also think it goes without saying that the only way to detect if any of this helps is having benchmarks that represent how your application behaves under real usage.
Providing zero cost abstractions is a goal of the Rust programming language. In this post we’ll explore the performance costs of various methods of indirection.
amdI recently ran some benchmarks on a Threadripper 3960X system and the results were surprising me quite a bit. Simplified, the throughput the benchmark recorded went down, from 341 MB/s on a MBP to 136 MB/s on a Threadripper desktop. Prior I had read Daniel Lemire's notes on the sub optimal performance for simdjson on Zen 2, which is heavily used in the benchmark, but the suggested drop were a few percent not half.
nodejsThe goal of this analysis is to try to understand what kind of gains a programmer should expect by using Rust and actix-web rather than Node and Express under typical use, without custom optimizations.
javascriptswc is fast. Very fast. It's 18x faster than babel on a single-core benchmark, and on a parallel benchmark, it's 68x faster than babel on a 4 core (8 HT) machine. Why? Just because it's written in rust? No. Its fundamental design differs from any other tool.
In Part 1 we looked at quickly doing operations on all items in a vector, using many CPU cores.
In this part we're looking at making it possible to update data in some other vector while traversing the first vector.
This is harder than it looks, since we can't just allow the parallel threads to update the auxiliary vectors. If we do, there will be races, unless we somehow know that different threads won't ever update the same item.
This blog post and git describes my search for a way to parallelize simulation of cars, intersections and roads in a computer game project. I won't be talking anything about the domain, but the problem is something like this:
There are three types of objects: Intersections, Roads, Cars.
Intersections have red lights, and alternately admit cars from different roads into the intersection. When a car arrives at its destination, the car object is updated changing its state from 'on road' to 'idle'.
The gist of it is that calculations are done for each intersection, and for each intersection the calculation may yield an update to a road or a car. There are hundreds of thousands of intersections, about the same number of roads, and a million cars. Calculations need to be quick, so we should run as parallel as possible.
I wrote down some details on the steps I take when optimizing the Rust compiler, using an improvement I just made to LEB128 reading/writing as an example.
Last week, Ibrahim Dursun published an article about zero-cost abstractions in Rust.
Unfortunately, except for a subpart of the article, this article did not reflect, in my own opinion, correctly what are zero-cost abstractions.Indeed, zero-cost abstractions, or “zero-overhead”, can be difficult to understand and to separate from other compiler optimizations, and can be easily misunderstood.
In this blog article, I discuss about this specific feature, and give you an example of how Rust is using it to deliver optimized code of your abstracted projects.
One of my colleagues was experimenting with Rust. He started by writing a sudoku solver which he has already written in C before. Once he was completed writing it in Rust, he was very disappointed because Rust version was twice as fast than the C version which was hand-optimised by pulling off all the tricks he knew to make it perform well. He eventually managed to make the C version as fast as the Rust version by removing the intrinsics.
This piqued my interest in looking into what kind of assembly code is being generated in the final binary.
One of my goals this year is to learn new things that take more than a few weeks to learn. I've been learning Rust. One of the claims I saw is that Rust's borrow mechanics allow it to optimize better than C++ does. I wanted to see this in action so I ran some simple examples through godbolt.
Ever since 2017 I have been writing my hobby projects in Rust. It’s not only the language which I fell in love with but also its speed. ❤️ Rust is known for its amazing speed. But, just because the language is fast does not mean all you apps will be as well. Here is my journey of how I sped up a program from running for over half an hour to only a few seconds.
wasm javaIn a previous post, we saw how Rust WebAssembly can be integrated into a JavaFX project using the Asmble tool. Here we look at an integration of the same functionality using the Java Native Interface (JNI). Finally, we compare the two approaches in terms of convenience and performance.
After reading the Cloudflare blog post on how to receive 1M packets per second, I wondered: How fast can we go with Rust and Tokio?
Rust is becoming a first class language in a variety of domains. At Discord, we’ve seen success with Rust on the client side and server side. For example, we use it on the client side for our video encoding pipeline for Go Live and on the server side for Elixir NIFs. Most recently, we drastically improved the performance of a service by switching its implementation from Go to Rust. This post explains why it made sense for us to reimplement the service, how it was done, and the resulting performance improvements.
Some time ago I had a fuzzy string matching problem to solve. I started looking for a performant Damerau-Levenshtein crate, and ended up writing my own collection of optimized edit distance algorithms, Eddie. In this post, using the lessons I've learned along the way and the Levenshtein algorithm as an example, I'll build upon a basic implementation step by step, and measure the results.
I did a line-for-line port of my eval library from Go to Rust, and right away it was 5x faster; I was pretty happy. But when I tried to further improve performance using techniques from other languages, it got slower... and the harder I tried, the slower it got! Rust performance was not intuitive to me.
Finally, after learning why my code was slow, I was able to boost performance 12000x, and my library was worthy of a new name: fasteval.
asyncNo one ever gets in trouble for posting micro benchmarks and making broad assumptions about the cause of observed results! This post will focus on a couple of such benchmarks pertaining to blocking operations on otherwise asynchronous runtimes. Along the way I’ll give only sparse background on these projects I’ve been working on, but plenty of links if you are interested in reading further. This blog post is sort of a followup to an URLO post: Futures 0.3, async♯await experience snapshot, and I’ll cross-post this one to URLO as well.
For the 0.2.0 release I was focusing on making rav1e faster. Luca Bruno asked me to give more context, this is the belated start of a set of blogposts about optimizing rust code.
unsafeIn this series so far, we've taken a C program and converted it into a faster, smaller, and reasonably robust Rust program. The Rust program is a recognizable descendant of the C program, and that was deliberate: my goal was to compare and contrast the two languages for optimized code.
In this bonus section, I'll walk through how we'd write the program from scratch in Rust. In particular, I'm going to rely on compiler auto-vectorization to produce a program that is shorter, simpler, portable, and significantly faster... and without any unsafe.
Can it be?
mutex spinlock(at least on commodity desktop Linux with stock settings)
This is a followup to the previous post about spinlocks. The gist of the previous post was that spinlocks has some pretty bad worst-case behaviors, and, for that reason, one shouldn’t blindly use a spinlock if using a real mutex or avoiding blocking altogether is cumbersome.
In the comments, I was pointed to this interesting article, which made me realize that there’s another misconception, "For short critical sections, spinlocks perform better".
Until today, I haven’t benchmarked any mutexes, so I don’t know for sure. However, what I know in theory about mutexes and spinlocks makes me doubt this claim, so let’s find out.
mutex spinlockIn this post, I will be expressing strong opinions about a topic I have relatively little practical experience with, so feel free to roast and educate me in comments (link at the end of the post) :-)
Specifically, I’ll talk about:
- spinlocks,
- spinlocks in Rust with #[no_std],
- priority inversion,
- CPU interrupts,
- and a couple of neat/horrible systemsy Rust hacks.
A seqlock — or “sequence lock” — is an optimized implementation of a reader-writer lock. In a seqlock “the data can be ‘protected’ by a sequence number. The sequence number starts at zero, and is incremented before and after writing the object. Each reader checks the sequence number before and after reading. If both values are the same and even, then there cannot have been any concurrent increments, and the reader must have seen consistent data.”
Let’s start with a skeleton of the implementation. This version has multiple problems and room for improvements, which we are going to explore next.
videoThe second official release of rav1e was focused mainly on speed. Later I'll write down what we used and what, in my opinion, are the best practices when you have to optimize a codebase like this one.
This is the third post in a series of posts exploring web services related technologies.
With the web service implementations in place, I evaluated them using an off-the-shelf benchmarking tool. This post documents the client-side observations from this evaluation.
Let's say that you've just updated the Rust compiler version and have tried to compile your application and see a failure that wasn't there before. That's likely due to a regression in the compiler. We've just released cargo-bisect-rustc, a tool that makes it super easy to find exactly when the regression happened.
This article presents a comparison of HTTP client apps in Node JS and Rust and looks at different aspects of those projects such as CPU and memory metrics, build time and distribution package size.
Thanks to PyO3, now writting a Python dynamic module in Rust is trivial and elegant. What you need to learn is the exmple on the README of PyO3 and rewritting the related function in Python to Rust. As a matter of fact, I firstly used rust-cpython, but I ran into some issues on macOS, hence I switched to PyO3 and it works smoothly.
Thanks to stewart/rff, the fzy Rust implementation is already good to go.
What I have to do is to wrap the Rust version fzy and replace the pure Python fzy in vim-clap with the generated Python dynamic module.
I did some tests laster, and found that the optimization result is very satisfying.
From pytest, Rust is 30x faster:
I last wrote in October about my work on speeding up the Rust compiler. With the year’s end approaching, it’s time for an update.
I have recently spent a lot of time writing pixel shaders and given that I have already written a pure Rust mod player I have started to think about trying my hand at writing a 64K intro in Rust.
One of the main challenges in writing a 64K intro is to squeeze all the code and assets into 64K of memory. There are several tiny frameworks written in C++ that set up a Windows app with a modern OpenGL context. I could not find anything similar for Rust so I decided to create the smallest possible bare bones app that does just that.
Today the language of choice for Machine Learning is Python (unless your working environment has some unusual constraints). I will take you on a journey. Hopefully, at the end of it, using Rust as a training backend and deployment platform will not look as crazy or confusing as it sounds. (Title aside, there is much more than speed to it)
It's been a while I have written a blog about the work I am doing lately. So yeah, I have been working on rav1e, the AV1 Encoder written in rust as part of Project Iris. Currently, if we see, there are other Open-Source Encoders available like libaom from Google, SVT-AV1 from Intel and Netflix. Rav1e’s memory footprint makes it a good starting point for new-cases like Software Encoding in ARM devices, Real-time streaming, while libaom and SVT-AV1 are either too slow or resource-intensive. So it would be much easier to make rav1e fast and power-efficient due to the low-complexity functions.
In the end, we are getting around ~12-20% Improvement in Encoding Time and FPS which is the first step making adoption of AV1 to Mobile devices. It is also very important to note that we’ve been using what SIMD code we have rather than proceeding in the order of lowest-hanging fruit. The relative gains would be more impressive if the outstanding functions were optimized first. Our priority was proving the infrastructure for merging, testing and benching on ARM Devices feasible and now it's more realistic.
With Moore’s law coming to an end, optimizing code to avoid performance pitfalls is becoming more and more useful. To this end, programming languages like Rust are designed to produce fast and memory-efficient programs out-of-the-box. When that is not sufficient, profilers like perf are useful to measure where the code is slow and therefore which algorithms and data structures should be optimized.
This experiment continues the work done in our pretend suite of microservices exposed via API Gateway to form an API with a code name of Slipspace in a mock company called STG. Slipspace drives are how the ships in the Halo universe travel so quickly to different sectors of the galaxy through something called Slipstream Space, so thought it was cool for a name requiring awesome warp API speeds.
In this tutorial, we will implement a Rust program that attempts to utilize 100% of the theoretical capacity of three relatively modern, mid-range CPUs. We'll use an existing, highly efficient C++ implementation as a reference point to compare how our Rust program is doing. We start with a simple baseline solution of 3 nested for-loops, and keep improving on the baseline solution incrementally, implementing 8 versions in total, until the program is going so fast it can hardly go faster. We'll approach the problem from the point of view of a C++ programmer who already knows how the reference implementation solves the problem, but is interested in an approach using the Rust language.
When writing a bump allocator, always bump downwards. That is, allocate from high addresses, down towards lower addresses by decrementing the bump pointer. Although it is perhaps less natural to think about, it is more efficient than incrementing the bump pointer and allocating from lower addresses up to higher ones.
It’s something of a meme lately to see whether your programming language of choice can take on the venerable wc, and what that might look like. The format seems to be: first do it simply, then idiomatically, and finally much faster. Of course, we’re not really “beating C” but rather “tackling a fun interview question in our favorite programming language.” My go-to these days is Rust, and since I’ve fielded the question of whether Rust is “my Haskell,” this all was too much to pass up. Let’s get started.
I got a project idea to test the feasibility of implementing Spark in a native language and if feasible, explore how efficient it can be in terms of performance and resource management. I know that Spark is heavily optimized over the years. I didn’t hope for any drastic difference in performance and if some difference is there, it most likely will be in RAM usage. Also, I want it to very general-purpose just like Spark. I decided to use Rust for the implementation.
So a couple weeks ago I was a little stung by the quote from This Week In Rust: “Rust compilation is so slow that I can fix the bugs while it still compiles the crates”. On the one hand, I have unfond memories of waiting for a Typescript project to compile, pack (aka link), minify (aka optimize), and so on, over and over, on every change. At least if it had been Rust I’d have been able to fix the bugs while it was doing this. On the other hand, it’s also mostly true: compiling Rust is heckin’ slow. So I’ve decided to dust off a backburner project for a while, and figure out just where rustc spends most of its time.
I have been running benchmarks of aggregate queries against the NYC taxi data set, using Apache Spark (JVM-based) as the baseline, since it is currently a popular tool for distributed compute, and a tool I am familiar with.
Since I do a lot of heavy numeric computation in C++ it was tempting for me to see how Rust compares in a shootout. I chose a floating point benchmark to implement in both languages in order to see the performance difference. I give commentary on why the performance is that way, and some potential fixes Rust could implement to close the gap.
async tokioWe’ve been hard at work on the next major revision of Tokio, Rust’s asynchronous runtime. Today, a complete rewrite of the scheduler has been submitted as a pull request. The result is huge performance and latency improvements. Some benchmarks saw a 10x speed up! It is always unclear how much these kinds of improvements impact “full stack” use cases, so we’ve also tested how these scheduler improvements impacted use cases like Hyper and Tonic (spoiler: it’s really good).
In July I wrote about my efforts to speed up the Rust compiler in 2019. I also described how the Rust compiler has gotten faster in 2019, with compile time reductions of 20-50% on most benchmarks. Now that Q3 is finished it’s a good time to see how things have changed since then.
Lately, there has been talk talk about improving build times, with a focus on reducing bloat like regex breaking out logic into features that can be disabled, cargo-bloat going on a diet, new cargo features to identify slow-to-build dependencies. The area that has been impacting me lately is build.rs. I've been code-generating compile-time hash tables (phf) which has added several dependencies to my build and takes a while.
Speeding up the Rust compiler isn’t the only way to make a Rust project build faster. Changing the crate structure of a project can also make a big difference. The good news here is that Eric Huss has implemented an amazing tool for visualizing Rust compilation, which can be used to identify inefficient crate structures in Rust projects.
Summary In a 45k LOC / 102-crate workspace, moving tests from member crates into a single workspace_tests crate achieved the following improvements:
Build and test duration in release mode reduced from 23 minutes to 13 minutes . Debug artifact disk usage reduced from 20 G to 7 G (65% reduction, fresh build), or 230 G to 50 G (78% reduction, ongoing development) Background The rate of software development is affected by many limits.
Cap'n Proto vs. Flatbuffers vs. Simple Binary Encoding
There’s a new hotness in performance measurements, and it’s called causal profiling. The idea behind it is that you want to measure how a speed up of a certain function would impact the runtime as a whole, which can be very counterintuitive in today’s multi-threaded world.
This blog post might interest three type of readers: people interested in tantivy: You’ll learn how tantivy uses SIMD instructions to decode posting lists, and what happens on platform where the relevant instruction set is not available. rustaceans who would like to hear a good SIMD in rust story. lucene core devs (yeah it is a very select club) who might be interested in a possible (unconfirmed) optimization opportunity.
Link time optimization (LTO) is LLVM's way of implementing whole-program optimization. Cross-language LTO is a new feature in the Rust compiler that enables LLVM's link time optimization to be performed across a mixed C/C++/Rust codebase.
A short story on how compiler updates can cause unexpected performance regressions.
I built a time tracking software named augr, and recently decided that it was time to make it faster.
For one of my projects, I need to use LLVM so I tried this cool inkwell crate that provides a mostly safe wrapper over LLVM. To my dismay, though, compiling this crate takes… a lot of time: Debug build: 1m 05s Release build: 3m 34s. By the way, I write this article for the sole purpose of trying to fix some problems there is in the crate ecosystem and by no mean I want to incriminate the author of this crate (or any other). I’ve been guilty of doing the same mistakes, but I learned from them and want other people to learn from them as well.
asyncIn Part 1, we covered how async fns in Rust are compiled to state machines. We saw that the internal compiler implementation uses generators and the yield statement to facilitate this transformation. In this post, we'll go over some subtleties that the compiler implementation must consider when optimizing generators. We'll look at two different kinds of analysis, liveness analysis and storage conflict detection.
I’m pleased to announce the release of Criterion.rs v0.3, available today. Version 0.3 provides a number of new features including preliminary support for plugging in custom measurements (eg. hardware timers or POSIX CPU time), hooks to start/stop profilers, a new BenchmarkGroup struct that provides more flexibility than the older Benchmark and ParameterizedBenchmark structs, and an implementation of a #[criterion] custom-test-framework macro for those on Nightly.
As you might have heard, async/await is coming to Rust soon. This is a big deal. Rust has already has popular crates (tokio, actix) that provide asynchronous concurrency, but the async syntax coming to stable in 1.39 is much, much more approachable. My experience has been that you can produce and reason about application flow much more easily, which has made me significantly more productive when dealing with highly concurrent systems. To kick the tires of this new syntax I dug into the nightly branch, and built a high-performance TCP client called clobber. In this post I'll talk about why I think async/await in Rust is a big deal, and walk you some of the code in clobber.
The issue to stabilize an initial version of async/await in Rust has left final comment period. The feature looks slated to stabilize in an upcoming release, most likely 1.39. One of the blockers mentioned in the RFC is the size of the state machines emitted by async fn. I’ve spent the last few months tackling this problem, and wanted to give people a window into the process of writing these optimizations, with all the intricacies involved.
I previously wrote about one period of improvement in Rust compiler speed. How are things going in 2019?
In one of my previous jobs I got a task: “For given image find popular colors in that image, so users can browse images by it’s colors”. This is where three languages comes to play. I have implemented histogram algorithm in C, Rust and Go.
I have written previously about my efforts to speed up the Rust compiler in 2016 (part 1, part 2) and 2018 (part 1, part 2, NLL edition). It’s time for an update on the first half of 2019.
Sometimes the question comes up about how CPU memory orderings work, and what they do. I hope this post explains it in a really accessible way.
Cedarwood is an effort to speed up jieba-rs, an efficient implementation of trie is needed in order to satisfying the following needs.
glam is a simple and fast Rust linear algebra library for games and graphics. mathbench is a set of unit tests and benchmarks comparing the performance of glam with the popular Rust linear algebra libraries cgmath and nalgebra. The following is a table of benchmarks produced by mathbench comparing glam performance to cgmath and nalgebra on f32 data.
The Computer Language Benchmarks Game is a free software project for comparing how a given subset of simple algorithms can be implemented in various popular programming languages. I converted the fastest (dating early 2019) n-body C-implementation (#4) to Rust (#7) in a one-to-one fashion, gaining a performance encreasement by factor 1.6 to my own surprise.
There are quite a few dimensions to how performance can vary between TLS libraries such as handshake performance and bulk performance. This series of blog posts measures and compares the performance of rustls (a TLS library in rust) and OpenSSL.
go pythonThis is a subjective, primarily developer-ergonomics-based comparison of the three languages from the perspective of a Python developer, but you can skip the prose and go to the code samples, the performance comparison if you want some hard numbers, the takeaway for the tl;dr, or the Python, Go, and Rust diffimg implementations.
This blog post is mainly to share my experience on taking an emerging programming language’s ecosystem seriously and evaluating it by working on a serious project, and see how far we can go in terms of performance and development experience. The project I chose as mentioned in the title is jieba-rs, the rust implementation of a popular Chinese word segmentation library: Jieba.
The two languages that I spent most of my time daydreaming about writing code in are Rust and Zig. Would the lack of features in Zig make me more or less productive than with Rust’s feature overload? Which language is more enjoyable to use for writing a small, self-contained computer graphics project? To find out, I decided to implement the same simple project in both languages: a small ray tracer, following the book Ray Tracing in One Weekend.
javascriptA blog about learning computer science concepts with practical projects
Today is an exciting point in the evolution of native GUI in Rust. There is much exploration, and a number of promising projects, but I also think we don’t yet know the recipe to make GUI truly great. As I develop my own vision in this space, druid, I hope more that the efforts will learn from each other and that an excellent synthesis will emerge, more so than simply hoping that druid will win.
In my work, I have come across a problem that is as seemingly simple, yet as difficult to get right, as making decent tea: handling smooth window resizing. Very few GUI toolkits get it perfect, with some failing spectacularly. This is true across platforms, though Windows poses special challenges. It’s also pretty easy to test (as opposed to requiring sophisticated latency measurements, which I also plan to develop). I suggest it become one of the basic tests to evaluate a GUI toolkit.
A detailed walk through how to memoize function calls in Rust.
For a recent personal project, I had only needed a fairly simple node.js server to do exponential and costly computing tasks. To be honest, I could have switched the entire tech stack, but I estimated that the development time of such a choice wasn’t worth it… Still, I had some functions taking ages to compute. So I had a look around, and decided to let that task be handled by a more appropriate language, in this case Rust.
Let me start by saying I really like Ruby. I tend to agree with the statement saying Ruby is optimized for developer happiness. However, nothing comes for free. Programming ecstasy is a double-edged sword and writing slow Ruby is as easy as it is pleasant.
Monomorphization has one problem (apart from being a ridiculous word that I’ll probably spell wrong every time): It generates rather a lot of code, bloating binary size and potentially pessimizing execution cache usage. Often, generics aren’t really needed for speed, but for ergonomics: Library code might want to present an easy-to-use generic interface that will automate some conversions. However, this often means that almost each user gets their own version of the code, leading to the aforementioned bloat (case in point: Earlier clap versions were notorious for adding hundreds of kilobytes to the binary size – for a simple command line parser).
Over the last year, the Backend Infrastructure team at Discord was hard at work improving the scalability and performance of our core real-time communications infrastructure. One big project we undertook was changing how we update the Member List.
As part of a project I’m working on, I sometimes find myself having to deal with quite large X12 files. What I’d really like is a small, self-contained tool that I can pass an X12 file to and rely on it to Do The Right Thing™ without any unnecessary incantations. Since I’m dealing with large source files it would also be nice if it was at least as fast as standard tools like sed. Sounds like a job for…
Since I last wrote about this topic, just only about a year ago select as used in the standard-library channel, has been deprecated. So it’s a good time to re-visit some of the concepts in that article, this time in the context of using crossbeam channels, and instead of using a made-up example, let’s dig into some real “production” code, as found in Servo. Let’s continue our exploration of Rust concurrency…
Majority of the people coming to Rust have C/C++ background which allows them to easily transition into Rust parallelism since it is so similar. However, for many people coming from other languages, it is a challenge. In this post, we will walk through the standard Rust parallelism tools as well as the motivation behind them. This will require a hardware deep dive at the beginning, followed by an explanation of the low-level tools, like atomics, and ending with an explanation of high-level tools like Mutex. Finally, we will explain how Rust guarantees safety in multi-threaded applications.
Over the past couple weeks I’ve been working on a couple different efforts around parallel query execution with DataFusion: 1. Benchmarking parallel query execution by manually creating one execution context per parquet partition and running on a thread, just to get an idea of expected performance, and comparing results to Apache Spark (running in local mode). 2. Creating a PoC of actual parallel query execution in the Arrow/DataFusion repository. This post is mostly about the first effort.
When optimizing code, one thing I’m always looking for is memory layout and access patterns. One such pattern is an arena: Reserve some sufficiently large space to put your objects in, then allocate by incrementing a pointer. If your objects are of a uniform type, you can basically simplify this to a Vec of that type.
I recently decided to learn more about Rust, and wrote a high performance RaptorQ (RFC6330) library. RaptorQ is a fountain code, and the core of the algorithm is a lot of matrix math over GF(256) – which translates into lots of XORs and reads from lookup tables. After getting the initial implementation working, I set about optimizing it. Below is a journal of the steps I took to profile and optimize the implementation.
With Rust, you can do low-level number-crunching and bit-by-bit processing, while enjoying memory safety and concurrency features. With Helix, you can use your Rust code inside of a Rails project.
I recently finished a detailed review of hashbrown, which will likely become the new implementation for rust's std::collections::HashMap. One of the most surprising things I found was in the implementation of insert. It was doing something that was so offensive to people who care about collection performance that we had designed an entire API to help people avoid it: it did two lookups in the map. However, after some more discussion and review, I concluded that this implementation was reasonable. This post will try to cover why that is.
I love Rust. But, as a data scientist, it’s still hard to use Rust on a daily basis. 90% of my programming these days is in Python.
My interest in Rust-based machine learning sparked several months ago. But, the key limitation I found was the lack of an ergonomic linear algebra library. There’s nalgebra and ndarray and a few others. Yet, I found none of them at the time ergonomic to work with, nor fast in comparison to writing the lower-level SIMD, BLAS, and Lapack code (I have picked up ndarray more in recent weeks and months).
While inconvenient, a few months later, I’m glad I had to take things a step further. Rust is great for writing performant code. The resources for writing quite low-level mathematics operations in Rust are quite good. Using blas-src and lapack-src, as well as Rust’s built in SIMD functions, we can write fast and surprisingly portable Rust code. You can even run Rust on the GPU using, at least, the same underlying code.
In this post I’ll talk about how to port a short raytracer written in C#/C++ codebase to Rust, then applying some simple optimizations by leveraging some features in Rust.
several models that make it easier to reason about concurrent programs have been envisioned over time. In this article, we'll have a quick look at a few of them, from new to not-so-new languages. I don't intend to give an extensive analysis of each solution, or make a formal comparison between them. My intention is to simply explain the basics of each solution and how they can be used in practice (with code samples that show off what the result of using the models might look like), so that other developers may have an easier time understanding them and deciding which solution, or language, might be better applicable to their particular problems.
A week or so ago, I saw the inferno project mentioned on the Rust subreddit. It was a rewrite of the great FlameGraph library into Rust. All of the work was being livestreamed by Jon Gjengset. I ended up watching some of the livestreams and had the idea of porting the stackcollapse-xdebug.php file to Rust, potentially so it could be included in the project in the future.
Algorithms and optimization to solve all Advent of Code 2018 puzzles in under one total second.
This is a follow-up post to Lock-freedom without garbage collection from 2015, which introduced Crossbeam, a Rust library that implements efficient lock-free data structures without relying on a tracing garbage collector.
Criterion allows you to benchmark against Rust stable, but it’s also providing a set of awesome features: Statistics: Statistical analysis detects if, and by how much, performance has changed since the last benchmark run. Charts: Uses gnuplot to generate detailed graphs of benchmark results. Stable-compatible: Benchmark your code without installing nightly Rust.
So, I have this STM32VLDISCOVERY dev board. It has the STM32F100RBT6B MCU, capable of running at 24MHz. On the board, there is a 8MHz crystal. Naturally, when you are new to microcontrollers (like me), you may have a few questions: When we upload a program on this development board, at what speed it is actually running? Is it using this external crystal? Why is this crystal 8MHz if the MCU is capable of 24MHz? If our program is not running at the maximum speed, how do we make it run at the maximum speed?
This post covers: Benchmark reports for contributors, Benchmark reports for users, Profiling with valgrind / kcachegrind, Reproducible benchmarks and graphics, and Tips for benchmark behavior and benchmarking other languages.
I’ve been getting into bioinformatics algorithms lately and ran across an interesting pull request that improved performance by changing a Rust match expression to a lookup. This felt quite surprising to me since, well, the match is so simple — why isn’t the compiler already generating optimal code for it?
You may have recently encountered and/or read this blog post criticising a possible C++20 implementation of Pythagorean triples using ranges. In it the author benchmarks different implemetations of the problem, comparing readability, compile times, run times and binary sizes. My main language these days is D, and given that D also has ranges (and right now, as opposed to a future version of the language), I almost immediately reached for my keyboard. By that time there were already some D and Rust versions floating about as a result of the reddit thread, so fortunately for lazy me “all” I had to next was to benchmark the lot of them.
For the first time, I took part in the Advent of Code this year. If you haven't heard of it, it's a daily programming challenge that can be solved in any programming language. Rust was very present in the Advent of Code community with people contributing a ton of Rust-related content. In the daily solutions thread on the /r/aoc subreddit, there were always several Rust solutions posted. Advent of Code really helps show off the things that make Rust shine, demonstrating the power and utility of many community-created crates as well as the language itself.
A gentle comparison between Rust & Python from multiple perspectives against a small, relatively simple problem.
I rewrote a Python project in Rust. The rewrite took a fair bit longer than expected, but the results were good (about 9 times faster and ½ the memory usage). In the process, I learned a fair bit about Rust.
Previously, I wrote about how Rust parsing is atypically slow comparing Rust's libcore implementation to a rudimentary parser I wrote. However, as others noted, the comparison was fairly limited. It didn't compare Rust's implementation to other implementations, such as glibc's strtod or Go's ParseFloat. The parser I implemented wasn't correct, it led to rounding error for most representations, by using floats for intermediate values. Furthermore, the comparisons used data unlikely to be encountered in real-world datasets, overstating the performance differences by forcing Rust to use slower algorithms. So, naturally, I aimed to address all these concerns. And finally, I forgot to disable CPU scaling, meaning CPU throttling could have led to inconsistent benchmarks.
A while back, there was a discussion comparing the performance of using the hashbrown crate (based on Google’s SwissTable implementation) in the Rust compiler. In the last RustFest, Amanieu was experimenting on integrating his crate into stdlib, which turned out to have some really promising results. As a result, it’s being planned to move the crate into stdlib.
While the integration is still ongoing, there’s currently no blog post out there explaining SwissTable at the moment. So, I thought I’d dig deeper into the Rust implementation to try and explain how its (almost) identical twin hashbrown::HashMap works.
A friend recently told me about a puzzle, which is a great excuse to explore programming craft. My Rust solution was a simple port of my second Clojure solution. The only major difference is that it takes advantage of mutability (which is idiomatic in Rust, unlike in Clojure). The Rust solution runs in about 4.22 ± 0.05 ms, or about 5x faster than the fast Clojure solution.
Niko Matsakis recently blogged about the Rust compiler’s new borrow checker, which implements non-lexical lifetimes (NLL). The new borrow checker is a really nice improvement to Rust, because it accepts many sound programs that the old borrow checker rejected.
Perhaps surprisingly, one of the most challenging things about operating RubyGems.org is the logs. A single day of request logs is usually around 500 gigabytes on disk. So every day, we generate about 500 files that are 85MB on disk, and contain about a million streaming JSON objects that take up 1GB when uncompressed. What we want out of those files is incredibly tiny—a few thousand integers, labelled with names and version numbers. Without any real idea of how to get those counts out of S3, I started by writing a proof of concept Ruby script that could parse one of the 500 log files and print out stats from it. Even on my super-fast laptop, my prototype script would take more than 16 hours to parse 24 hours worth of logs.
Recently I have been working on Rust datastructures once again. In the process I wanted to test how my work performed compared to a standard library RwLock and Mutex. On my home laptop the RwLock was 5 times faster, the Mutex 2 times faster than my work.
So checking out my code on my workplace workstation and running my bench marks I noticed the Mutex was the same - 2 times faster. However, the RwLock was 4000 times slower.
pythonRecently a colleague of mine told me about a small bottleneck with url quoting since we are quoting a lot of storage keys at least once when loading or storing a dataset. To speed it up, we are going to write a C-Library in Rust and invoke it from Python.
When I first started building the dtparse crate, my intention was to mirror as closely as possible the equivalent Python library. Python, as you may know, is garbage collected. Very rarely is memory usage considered in Python, and I likewise wasn’t paying too much attention when dtparse was first being built.
This lackadaisical approach to memory works well enough, and I’m not planning on making dtparse hyper-efficient. But every so often, I’ve wondered: “what exactly is going on in memory?”
But today (October 4th, 2018), the pest website featured a very misleading graph. Yes, a pest 2.0 parser that does not convert the input to Rust types is indeed faster than a nom 4.0 parser that does convert the input to Rust types. But what happens if I write a nom 4.0 parser that does not convert its input to Rust types?
Rust makes writing parallel code safe. Rayon makes it easy.
I reimplemented a body of C software in Rust, and it performed better for the same task; what’s going on? And is there anything broader we can say about these results?
To explore this, I ran some statemap rendering tests on SmartOS on a single-socket Haswell server (Xeon E3-1270 v3) running at 3.50GHz. The C version was compiled with GCC 7.3.0 with -O2 level optimizations; the Rust version was compiled with 1.29.0 with --release. All of the tests were run bound to a processor set containing a single core; all were bound to one logical CPU within that core, with the other logical CPU forced to be idle. cpustat was used to gather CPU performance counter data, with one number denoting one run with pic0 programmed to that CPU performance counter. The input file (~30MB compressed) contains 3.5M state changes, and in the default config will generate a ~6MB SVG.
lolbench compiles ~350 benchmarks with every Rust nightly. It then runs them and highlights potential performance regressions in the standard library and the output of the compiler. Each toolchain’s run is summarized with a list of likely candidates, as seen in the image below, and we’re now getting started using these to safeguard the performance of Rust programs. Come help!
Today I released ppbert 0.8.4. This release also marks the first time that one of my original test files can be pretty printed in less than a second. I’ll use this occasion to look back on ppbert and how I was able to improve its performance, little by little.
Alacritty, the OpenGL terminal emulator written in Rust, now supports scrollback! Performance has improved, and we've got benchmarks to share.
For a small side project I’m working on, I’m using a Sudoku puzzle solver and puzzle generator that I’ve written in Rust. The experience was fun, so I thought I’d write up a little bit about the algorithm I’ve used and some interesting stats about how it performs.
Rust is all about paying only for what you use, and gives us plenty tools to eliminate unneeded allocation. One of the tools that is used in a lot of crates (crates.io shows 98 dependent crates) is SmallVec. It is also used in the Rust compiler. I recently got around to speed up the operation of getting a SmallVec from a slice of copyable data. In short, they’re awesome.
I already covered some inner-loop optimization tricks for low-level Rust code in mtpng, but how do you check how fast bits of your code are anyway?
The way to go is to use a sampling-based profiler native to your operating system. I’ve done most of my detailed profiling on Linux, using the “perf” tool.
In my last post I wrapped up the patches to improve perceived performance of screenshots on the Linux GNOME desktop. With that done, why not implement my crazy plan for parallel PNG encoding to speed the actual save time?
It’s been a while since I’ve been playing the benchmarksgame with Rust. But I recently found an interesting crate called packed_simd which had a SIMD-ified version of some benchmarks, so as Rust stable now has stdsimd, we should be able to speed up our benchmarks quite a bit.
Aim: Measure how fast a fetch from L1 cache is when compared to a fetch from memory. Instead of writing pure assembly code, we will use Rust's inline assembly feature.
I set out out my goal 9 for Rustnish: Write benchmark code that compares runtime performance of Rustnish against Varnish. Use cargo bench to execute the benchmarks.
The basic idea of a performance test here is to send many HTTP requests to the web service (the reverse proxy in this case) and measure how fast the responses arrive back. Comparing the results from Rustnish and Varnish should give us an idea if our performance expectations are holding up.
This document is a compilation of various benchmarks and comparisons between code counters, namely tokei, cloc, scc, and loc. This document seeks to compare performance, and accuracy of the code counters. polyglot is not currently included as it was unabled to be installed on the machine at the time of writing.
We leverage the elegance of kdb+ and the power of Rust to create data applications that can process data at the rate of tens of GB/second on consumer grade hardware.
With the latest release of 1.27 of Rust (SIMD support) the code counters written in Rust were suddenly a lot faster in Linux. In fact it meant that the fastest one tokei was suddenly faster than my scc for almost all tests. In addition a new project polyglot written in a language I have never heard of ATS popped up which is also now faster than my Go program for any repository when running on a machine with less than 8 cores.
Rust 1.27.0 has brought SIMD (Single Instruction Multiple Data), also known as vectorization, to stable Rust. If you read the announcement, you will see that SIMD should bring performance enhancements to our applications if we learn how to use it properly. But, for that let's first dive into how SIMD works.
In my last post on optimising my Rust path tracer with SIMD I had got withing 10% of my performance target, that is Aras’s C++ SSE4.1 path tracer. From profiling I had determined that the main differences were MSVC using SSE versions of sinf and cosf and differences between Rayon and enkiTS thread pools. The first thing I tried was implement an SSE2 version of sin_cos based off of Julien Pommier’s code that I found via a bit of googling. This was enough to get my SSE4.1 implementation to match the performance of Aras’s SSE4.1 code. I had a slight advantage in that I just call sin_cos as a single function versus separate sin and cos functions, but meh, I’m calling my performance target reached.
The other part of this post is about Rust’s runtime and compile time CPU feature detection and some wrong turns I took along the way.
Since my last post, rustc-perf — the benchmark suite, harness and visualizer — has seen some improvements. First, some new benchmarks were added: cargo, ripgrep, sentry-cli, and webrender. Also, the parser benchmark has been removed because it was a toy program and thus not a good benchmark.
Following on from path tracing in parallel with Rayon I had a lot of other optimisations I wanted to try. In particular I want to see if I could match the CPU performance of @aras_p’s C++ path tracer in Rust. He’d done a fair amount of optimising so it seemed like a good target to aim for. To get a better comparison I copied his scene and also added his light sampling approach which he talks about here. I also implemented a live render loop mimicking his.
It’s often said1 that the slowest code is that which has been optimised without benchmarks. You wouldn’t expect your code to work if you never ran it, so why should you expect it to be fast if you never benchmarked it? Writing good benchmarks is a bit of an art, because it’s really easy to accidentally write benchmarks that make your code seem fast, when really the compiler is applying some optimisations that work in the side-effect-free world of the benchmark but can no longer get applied when you put it out into the wild.
A few weeks ago, I set out to convert bytecount’s benchmarks to criterion, a statistics-driven benchmarking framework started by Jorge Aparicio and maintained by Brook Heisler.
Before, bytecount used bencher for its benchmarks, which is a straight port of the unstable, nightly-only std::test benchmark framework, extended to work with stable Rust. This was a great benefit compared to std::test, because now we could benchmark on all Rust versions (stable, beta, nightly, some specific version) without needing to fear regressions.
smallvec is a library by the Servo team for reducing the number of allocations for dynamic arrays in the case that most of those arrays are below a certain size. Because malloc is fast, for many cases it’s actually slower to use SmallVec than just using Vec because the one-time cost of the initial allocation is dwarfed by the lifetime cost of SmallVec’s increased complexity. You can see that switching to Vec actually improves speed on many of SmallVec’s own benchmarks.
TL;DR: The Rust compiler has gotten 1.06x–4x faster over the past month.
Recently, a benchmark made it to the top of /r/programming, featuring Rust among other languages, and I was a bit surprised to see that the idiomatic Rust program was not competitive with the best-tuned C++ solution. The benchmark implements a binary tree, and the C++ solution leverages raw pointers while Rust would use an Option<Box<Node>> to represent its tree. Since Option knows that Box is non-nullable, it should compile down to a raw pointer. Quickly inspecting the Rust and C++ versions would not let me find where the performance difference came from.
One of Rust’s design goals is to be fast. That actually needs two distinct things from the language. First, is it shouldn’t introduce too much (preferably zero) overhead for its abstractions and be fast out of the box. Many people coming from the high level languages (python, javascript, …) find this to be the case ‒ just type the program, compile it (with --release) and it’s reasonable fast. The other, no less important, is allowing the programmer to tweak some knobs when trying to squeeze a bit more speed out of the program.
I’ve decided to test the second a bit and see how far I could go. I’ve chosen matrix multiplication as a case study, for several reasons. I’ve played with it before (in my master’s thesis), it’s relatively simple and the effects of optimizing it can be great. For simplicity, I’ve decided to multiply only square matrices with power-of-two sizes, but these restrictions can be lifted in a real implementation without significantly loosing performance ‒ only the code gets somewhat more complex and hairy.
I’d like to share a quick story about the sheer power of LLVM and the benefits of using higher-level languages over assembly.
Once upon a time, I wrote an interpreter for Stratego Core in Rust, which I named strs. Stratego Core is the core language that Stratego is compiled to before the compiler goes further (to Java, or previously to C). A core language is an intermediate representation that is a subset of the surface language.
While I optimised that interpreter quite a bit, I noticed that the CTree (Stratego Core Abstract Syntax Tree) that the compiler spit out for me to interpret was very unoptimised. Therefore one the plans I described at the end of the blog post was a little tool for Copy Propagation on CTree files. This post is about that tool, and the optimisations in the interpreter that made it obsolete again.
Seeing Nick Nethercote’s blog post about speeding up the compiler, I started wondering just how fast could a Rust compiler be? How fast could we compile a simple example? How fast can we compile a Rust hello world?
18 months ago I wrote about some work I did to speed up the Rust compiler (rustc). I’ve recently taken this work up again. Also, in the meantime rustc’s build system has been replaced and its benchmark suite has been overhauled. So it’s a good time for an update.
A few months ago, Bünz, Bootle, Boneh, Poelstra, Wuille, and Maxwell published Bulletproofs, which dramatically improves proof performance both in terms of proof size and verification time. In addition, it allows proving a much wider class of statements than just range proofs.
At Chain, we (Henry de Valence, Cathie Yun and Oleg Andreev) have been working on a pure-Rust Bulletproofs implementation, whose initial version we are publishing today, together with a set of notes.
This new version comes with great performance improvements. We're talking about 3x faster on macos, 2x faster on linux and 3x faster on windows (the benchmarks are at the end of the post).
For one of our customers at Centricular we were working on a quite interesting project. Their use-case was basically to receive an as-high-as-possible number of audio RTP streams over UDP, transcode them, and then send them out via UDP again. Due to how GStreamer usually works, they were running into some performance issues.
This blog post will describe the first set of improvements that were implemented for this use-case, together with a minimal benchmark and the results. My colleague Mathieu will follow up with one or two other blog posts with the other improvements and a more full-featured benchmark.
The short version is that CPU usage decreased by about 65-75%, i.e. allowing 3-4x more streams with the same CPU usage. Also parallelization works better and usage of different CPU cores is more controllable, allowing for better scalability. And a fixed, but configurable number of threads is used, which is independent of the number of streams.
Writing a debugger for C++ on Linux, you spend a lot of time examining pretty-printed DWARF debug information using tools like readelf, objdump or dwarfdump. Unfortunately this can be quite slow.
I decided to try to speed dwarfdump up. TL;DR: I reduced the dump time from 506s to 26s by fixing some simple issues and taking advantage of Rust "fearless parallelism". I think there are interesting opportunities for speeding up many kinds of command-line tools using Rust and parallelism.
Blazing fast, low requirements, computationally intensive operations on Node.js using Rust
When I built Finda, I wanted it to be fast — specifically, to respond to all user input within 16 milliseconds.
Given this goal, you might be surprised to learn that Finda is built with Electron, a framework that’s often decried for being the opposite of fast.
Recently, I came across an ad for a job that had a precondition for application: it required you to first solve a ✨programming challenge✨:
benchmarkingCriterion.rs is a statistics-driven benchmarking library for Rust. It provides precise measurements of changes in the performance of benchmarked code, and gives strong statistical confidence that apparent performance changes are real and not simply noise. Clear output, a simple API and reasonable defaults make it easy to use even for developers without a background in statistics. Unlike the benchmarking harness provided by Rust, Criterion.rs can be used with stable versions of the compiler.
The story about Rust’s async is still a bit in flux. There’s a bunch of libraries with their pros and cons and different approaches. Even I’m a bit to blame for that, as I’m writing one of my own, called Corona.
faster began as a yak shave, created to aid base💯 in its quest to become the fastest meme on Github. Writing an explicit AVX2-accelerated version of base💯's encoder and decoder, then realizing I'd have to do the same thing again to see the speedups on my Ivy Bridge desktop, pushed me to make this library. Months later, it has blossomed into its own project, and has eclipsed base💯 in both popularity and promise.